Josh Scannell | What Can an Algorithm Do?
Keywords: DAS, data issue, IBM, Josh Scannell, neoliberalism, NYPD
Proactive policing and the NYPD’s DAS system
“The breach of the techno-civilized logic of computation and calculations could thus be argued as madness itself.”
– Eyal Weizmann, The Least of All Possible Evils (16)
“If plantation slavery resides at the origins of capitalism, this is because the plantation-form insistently presides over those moments in which capitalism re-originates itself, moments in which new epochs of exploitation and accumulation emerge – early industrialism, Fordism, post-Fordism. Every form of capitalist labor process bears a homology to the plantation, because the plantation is all there is.”
– Chris Taylor, “Plantation Neoliberalism”
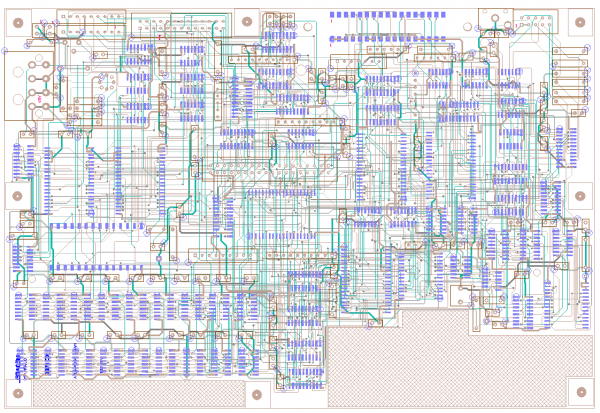
Much of the discussion surrounding emerging surveillance technologies and large-scale data processing systems addresses questions of definition (“what is big data?”) and novelty (“is big data more than just techno-branding?”). Rather than interrogate whether critics understand what they mean when they talk about algorithms or “big data,” I focus instead on the political aesthetics of governance that many of these systems crystallize. Yet what is often missed in debates surrounding this algorithmic turn in governance is that the relative effectiveness of these systems’ delivery on promises is borderline-irrelevant. To paraphrase Deleuze: we ask endlessly whether algorithmic data analytics systems are good or bad, are novel or merely digital hype, but we rarely do we ask what an “algorithm” can do.
First, in asking what an “algorithm” can do, I am by no means posing a technical question. An algorithm is a series of instructions; asking what one “can do” in any general sense is rank absurdity. Instead, I consider the algorithm as a political object, as an assemblage of forces that imprints itself on the social as something like “algorithmic governance.” Intrinsic to the design of an “algorthim” are decisions that, when routed through the technocratic administration of computation, transform from ideological commitments into material accounting.
Second, by situating the “algorithm” as a political object, I am demanding that politics and aesthetics be understood as inseparable. This is true, not only in the sense that aesthetics are mobilized in the service of politics, or that regimes of the sensible triangulate (Rancière) the political imaginary, but also in that the entirety of the social field is an aesthetic project. The algorithm, as that which makes sense of quantified sociality, is thus first and foremost an aesthetic machine.
Third, “algorithms” are material and real social processes. The “algorithm” is not an idealization, a linguistic tool, or an emergent dispositif. Instead, it collects under its anima concrete materialities. Undeniably, the “algorithm” bends historical materials towards its service. For instance: in a weird hybridization of post-industrial neoliberalism and 19th-century railway-baron plutocracy, fiber optic cables run alongside rail lines, taking advantage of eminent domain. Exploiting legal statutes designed to proliferate the arterial rail infrastructure of American empire, “the algorithm” transforms defunct rail links like Council Bluffs into Google server farm hubs. Rather than residing in an immaterial cloud, big data circulates through two centuries of accumulated capital geographies. Google’s famed search algorithm owes as much to 19th-century railroad engineers and 20th-century American industrial collapse as it does to 21st century computer science engineers and venture capitalists.
Fourth, at the same time that “the algorithm” actively mobilizes concrete social relations, it occludes these relations by reformatting what qualifies as the social. In its technocratic utopianism, data analytics systems render multidimensional processes into numbers subject to mining, dependent upon a logic of smoothness in order to function. This necessarily reduces the complex social world into terms of calculation and irruption that can only be understood by machines. Structural inequalities become computational errors and inefficiencies. Labor is not so much reified as rendered invisible by mathematics.
Clearly, then, when speaking of “the algorithm,” we are not speaking of algorithms per se, but rather of a shift in governmentality catalyzed by data analytics technologies. What, then, can an algorithm do? Let us take as our example the Microsoft’s Domain Awareness System, which has been in operation in New York City since 2012. Built for the NYPD as a crime prediction platform, the DAS does not work to predict so much as to enshrine contested neo-plantation systems of rule as technocratic abstractions, infrastructural, and inevitable.
II.
Reactions to new data mining systems typically present in three distinct forms. The first “critical” form decries digital surveillance as a further step towards an Orwellian dystopia. The second “liberal” form argues that new technologies merely make existing information more efficient in order to better provide services. In the case of marketing, for instance, this translates into personalized advertising. In the case of policing, it is presented as honing the ability to anticipate crime outbreaks, on the one hand, and reducing human error on the part of individual officers—though rarely for reducing institutional discrimination—on the other. The third, “technoskeptic” form generally argues that these systems are mostly hype, do not work very well, and are novel neither in concept nor execution.
Each of these positions came into focus when the NYPD rolled out DAS in 2012. Plenty of headlines referenced Big Brother (in no small part due to its downright dystopian moniker); plenty of liberal commenters said that this was a good way of addressing prejudices within the department and efficiently utilizing police resources; plenty of critics rightfully pointed out that this wasn’t going to look any different for people on the street. This was, after all, at the height of the NYPD’s use of “Stop, Question and Frisk,” a policy so blatantly racist that the federal government was forced to declare it unconstitutional.
But while each response has a degree of merit, most miss critical points. Let’s start with the punch line: predictive crime software has nothing to do with preventing crime. Instead, it simultaneously treats public order clinically, in the vein of disease prevention or weather prediction (whose algorithms form the basis of much of today’s crime prediction software) and legitimates plantation neoliberalism and heterosexist ideology as the base-line measurement of what a city should be. Both moves are driven by a mathematization of aesthetic politics, as a reorganization of ontology into computation.
While weather and disease outbreaks are hardly “natural” phenomena, crime is a particularly bizarre social process to imagine as computational. Crime, after all, is radically unstable and fluid – both over the longue duree and in the more quotidian time scales of policing. Inherently political, crime is a social and historical process of constant redefinition and contest. Even supposedly immutable prohibitions, such as murder, are radically contingent on circumstance, evidenced nowhere more so than by the recent national series non-indictments against murderous police officers.
When we consider the overall calculus of what constitutes “crime,” however, the overwhelming majority of criminal acts are the sorts of property and quality-of-life violations that are essentially at the definitional discretion of the police officer to produce. In other words, crime does not exist without the police. Police officers, in a literal sense, produce crime by recognizing prosecutable violation to the state. Officers, after all, have to file charges for a crime to have been committed.
This process does not emerge ex nihilo, but instead out of a state strategy in which the contours of livable life are violently drawn by carceral apparatuses interested in maintaining order. The DAS reimagines this inherently violent relationship as something more akin to state-sociology, in which police officers operate as data collectors, providing the raw material for Microsoft’s number crunchers to build “heat maps” of “hot spots.” Police no longer protect and serve a citizenry, but rather become agents in the clinical epidemiologization of crime.
In transmogrifying state violence into the patrolling of rationally predicted outbreaks, “proactive policing” becomes more palatable to a citizenry that might otherwise have moral or political concerns over incessant harassment, especially of the non-normative (read: not white, not hetero, not moneyed, not cisgender). In reducing the mess of social contest to “clean” computation, predictive analytics systems seek to eliminate the social from sociality—an aesthetic decision.
Of course, aesthetic decisions have historically been inherently political. Broken Windows policy, for example, imagined the ideal city as a heteronormative, white space in which bodies cycle between discrete private spheres, and capital flows are unimpeded by human obstructions. Police strategies, such as charging non-white bodies present in public spaces with offenses such as obstructing street traffic or failure to promptly present identification, are designed to erase non-white bodies from the ideal city. The city’s 1990s campaign to “clean up” Times Square eliminated spaces of non-heteronormative sexuality to make way for Disney. The possession of city-issued condoms was criminalized in a broader effort to erase those whose presence offend the ideology of the ideal city. In a post-industrial economy, we witness victims of capital restructuring subjected to a heightened vulnerability to arrest, and even murder, for resorting to informal means in order to eke out a living (evidenced by the recent strangling of Eric Garner for selling loose cigarettes). Outlawing tagging and other means of marking social space criminalizes even symbolic resistance to gentrification and the wholesale dispossession of the city’s working class. The city’s oppressed class is expected to live in private homes, be docile and quickly moving in public, to endlessly cycle between jobs that do not afford a life in the city, to passively obey public authority—ultimately, the entire city is expected to live in imitation of privileged whites. This is the logic of Jim Crow. It is the logic of the plantation. It is also the logic of the present.
With predictive analytics, a model city with zero criminal outbreaks is heralded as a computational, and thus a modern police, goal. The biopolitical prosecution of broken windows is the baseline logic of the Domain Awareness System. It generates the data converted by the analytic system into hot spots, supposedly to aid police officers, in real time, to know where crime is likely to occur. But a heat map is nothing more than the aesthetic logic of our state and civil society’s image of the ideal city. It is ultimately an aesthetic logic of erasure.
Turning police officers, and surveillance apparatuses more generally, into data collection agents programs these systems to reproduce structures of inequality and violent oppression. Rendering racial capitalist police strategies as neutralized “heat maps” of probable criminal activity neutralizes the carceral state’s contestable work into a denuded mathematical simulacra of a white supremacist fantasy. This is then perversely sold to the public as an efficient means of eliminating problems of bias and resource waste from the necessary work of policing.
This logic of algorithmic-driven human resource distribution is a form of “deskilling,” in the sense that the major decisions about how and where policing should be done are withdrawn from human agency. Moreover, it severs the relationship between the police and their communities. After all, the stated logic behind this sort of system is to transform policing into data entry. I believe we should call it what it is: a hyper-rational way of building plantation capitalism into the city’s electronic infrastructure. Capital and governance finally found a way to make Jim Crow “disappear,” while continuing to pursue it as a matter of public policy. As Ruth Wilson Gilmore put it: “capitalism requires inequality; racism ensures it.”
III.
In the same year that the NYPD and Microsoft debuted the Domain Awareness System, IBM (Microsoft’s main competitor in analytics crime-prevention software) released a TV spot called “Predictive Analytics – Police Use Analytics to Reduce Crime.” The following is the voiceover, spoken from the perspective of a white police officer cruising around an LA-looking urban sprawl:
“I used to think my job was all about arrests. Chasing bad guys. Now I see my work differently. We analyze crime data, spot patterns, and figure out where to send patrols. It’s helped some US cities cut serious crime by up to 30% by stopping it before it happens. Let’s build a smarter planet.”
The advertisement follows two characters, both white, early middle-aged, male. The officer speaks in a region-neutral, soothing (almost affectless) cadence over shots of him casually paying for coffee at a diner, driving down nearly empty freeways and suburban back roads. Interspersed are images of a man we are implicitly led to understand as the criminal—or, to use the parlance of the text, the “bad guy.”
They seem to coexist as some sort of dystopic odd couple. The officer drives smoothly, glancing casually at his in-car computer, while the “criminal” screeches around corners and glances furtively at his watch. In the last ten seconds of the spot, there is an establishing shot of a 7-11 style market, “Remo’s”. The “criminal” pulls his beater into an empty parking space, then animatedly pulls gloves over his hands. As he turns the corner to enter the store (presumably to rob it), he sees our officer, pleasantly sitting on the hood of his cruiser, smiling and drinking his coffee. The officer raises his cup in greeting and smiles a knowing, polite smile. The “criminal” sighs, turns around and walks back to his car. No words or suspicious looks are exchanged. This is a familiar dance for these two, a relationship so routinized that fails to provoke discourse. As the “criminal” calmly walks away, the IBM logo fills the screen.
The logic of the DAS parallels IBM’s data analytics ad. The mutual production of the metaspace of databanks and the physical space of the built environment route through one another, producing a metastability that drives the generation and circulation of capital (Thrift 2008). The same movement that isolates populations from circulations of capital and legitimacy (read: the structurally disadvantaged) digitally commodifies that same population’s isolation and oppression. Here, IBM reads the “aquatic relations” of the wretched of the earth and preemptively translates them as disorder, ripe for commodification through the circulation of data-driven population “knowledge.” (McKittrick and Woods 2007).
Rather than see proactive policing as intrusive or violent tactic of rule, IBM casts it as an inevitable, logical outgrowth of data collection that eliminates the “need” for violence. Or, as Mark Cleverley, IBM’s Director of Public Safety aptly put it, “historically policing has been involved with trying to ‘do better’ the job of reacting. What people are starting to do now is a new approach from getting better at reacting to getting better at predicting and becoming proactive.” The translation? We have mathematized Broken Windows.
The challenge of spots like IBM’s is to cast these cases of predictive analytic policing as commonsensical and dispassionate. To maintain this imagined semiosis of temporal violence, the ad must deny the reality of the very structural conditions that produce crime and criminality in the first place. Although IBM claims, elsewhere, that its analytics of public safety are simply advancing our understanding of urban geographies, in their public presentation, urban geography and its concomitants such as wealth inequality, racial oppression, gender oppression, and differential access to public services, are (quite literally) “whitewashed.” Although there is some visual indication of class differential between the lawman and the lawbreaker, the urban worlds they coast through are undifferentiated. The city becomes a network of neutralized, empty, racially homogenous highways that mimic the lines and contours of a microchip. Crime control appears here like some sort of gentleman’s agreement between white lawmen and white lawbreakers, rather than as the violent imposition of heteropatriarchal racial capitalism on the surplus populations of neoliberal urban fantasias.
This refined fantasy of the city– where time itself is conquered by the futurity of software–is the basic intellectual model upon which IBM is dependent. Explaining their business project elsewhere, they claim that “with the ability to sense and react, and even predict, cities around the world are getting smarter and getting results.” IBM sees the city as a neutral intelligence whose data generation merely needs networking. To that end, they have tellingly rationalized their smarter cities project into eleven components: traffic, energy and utilities, retail, healthcare, airports, social services, communications, education, rail, public safety, economic development.
For IBM, the distinctions between these branches are merely technical, rather than unique fields of production, dissemination, and consumption. IBM’s promotional material presents a uniform aesthetic and logic that flattens these supposed core functions of the city into ontologically equivalent data generation components. Under such a system, their linking feature is not their spatiality but, on the contrary, their capacity to be abstracted into data relations. IBM’s mission is, first, to institute mechanisms by which it can constantly be collating and analyzing data in real time and, second, to make all that data mutually constitutive and predictive. There should be no analytic difference between data projects measuring and predicting crime and public safety, traffic flows and retail. Rather, it is this precisely this interchangeability that IBM is monetizing. IBM is depending on the emergent nature of recombinable data not to reflect the city but to refine it. Their model is to produce marketable bodies of data, obscuring the distinction between human and non-human, effacing the physical and the immaterial, datalogical and algorithmic, into a vortex of emergence and capturability.
The consequence of “whitewashing” data collection obviously materializes in the policing of the “real world.” IBM claims that their data analytic programs helped reduce crime in Memphis by over 30%. Microsoft, with the NYPD, hopes that the Domain Awareness System’s capacity to do things like digitize and compute bodily radiation levels and human spatial mobility will effectively nullify the emergence of criminal behavior. Every time a body is stopped and frisked by the NYPD, the relationship that is enacted is not a one-to-one, but also a production and performance of data, virtualizing the dissolving and dangerous body of crime into a graspable and controllable horizon of the real. These spectral data bodies are not preempting the real; they are actively producing the real. Data is neither representational nor hauntological (Derrida 2000), it is ontogenetic.
The NYPD’s Domain Awareness System, like IBM’s Smarter Planet, points toward a fantasy of smooth processes and processing. Gone are the messy histories and tragedies of urban contest that are registered as criminal. Gone are the difficult operations of adjudicating law through the inherited subjectivities of race, class, and gender. Instead we have a futural game of cat and mouse where the cat is cybernetically enhanced to predict the mouse’s every move. For this reason, the characters must become generic representations of entire power structures: in the logic of IBM, all such power structures are reducible, recombinable, and predictable. The city and its contestations are merely so many points on a data map. The data and the algorithm produce their own ontological conditions of emergent possibilities that are viral and positive rather than representational and negative. The algorithm is the apparatus that organizes and activates the necropolitical logic.
This turn towards the parametric and the algorithmic in the modeling of futural terror/crime based on available crime statistics links programs like IBM’s public safety analytics to weather prediction programs, as well as digital architectures for predicting the emergence of disease (Parikka 2010). The database, in this light, becomes a technical tool by which to assemble “dangerously” emergent, entangled, demi-human populations, and to flood them preemptively with the tools of the carceral state (police, insurance, health care, carceral education, foreclosure, limited credit, structural poverty, etc.). This assemblage produces a burgeoning plane of incarceral data, ripe for monetization and reduced agency.
Big data functions precisely because the personal data trails and tracings of any individual subject are networked into a sort of dark web of temporally deep and spatially unbounded sets of resonances and patterns. Personal data traces are only valuable to big data in so far as they are enmeshed within diffuse and complex systems with unclear affinities. And it is precisely the proprietary capacity of developed algorithms to make sense of this dark web that renders it monetizable.
Rather than predicting the emergence of human activity, big data’s computational intelligence is predicated precisely on provoking the emergent (hence spokespersons for the DAS perpetually reminding us of the capacity to produce geospatially “accurate” maps of past and probable future sites of criminal/terrorist activity). In the world of big data, the indetermination that is at the root of the socially constructed subject or independent agent is already given over to metrics of prehension, which sociologists have long seen linked to neoliberal chains of capital production and circulation.
This is precisely the point. The IBM predictive analytics ad shows us not a cop predicting crime, but rather the police officer is exploiting a pre-existing spatialized relationship of generalized insecurity and criminality (implicitly deracialized). But what IBM and Microsoft are banking on is us not being able to tell the difference.